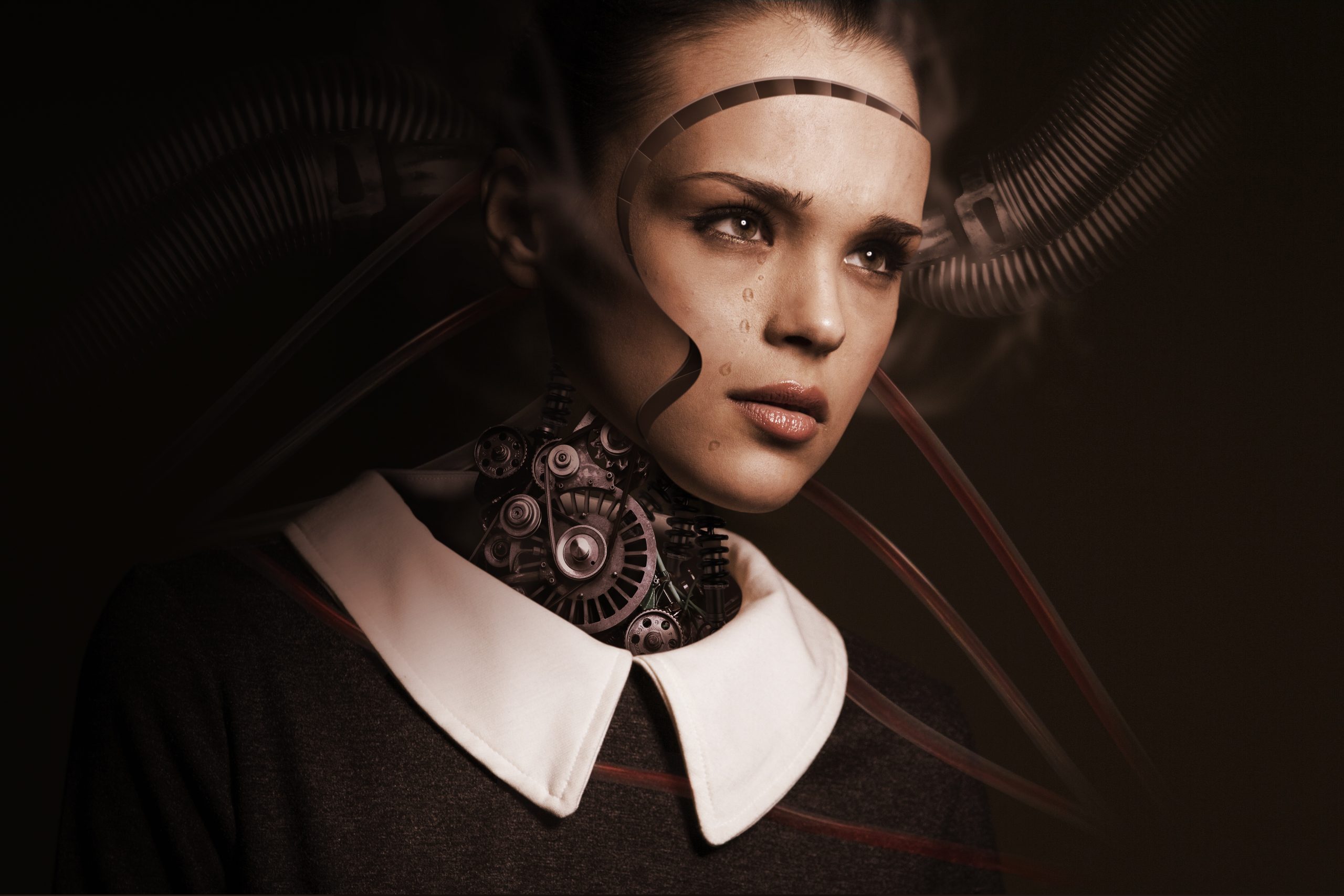
It’s going the distance — DeepMind breaks 50-year math record using AI; new record falls a week later AlphaTensor discovers better algorithms for matrix math, inspiring another improvement from afar.
Benj Edwards – Oct 13, 2022 8:46 pm UTC Enlarge / A colorful 3×3 matrix.Aurich Lawson / Getty Images reader comments 80 with 51 posters participating Share this story Share on Facebook Share on Twitter Share on Reddit
Matrix multiplication is at the heart of many machine learning breakthroughs, and it just got fastertwice. Last week, DeepMind announced it discovered a more efficient way to perform matrix multiplication, conquering a 50-year-old record. This week, two Austrian researchers atJohannes Kepler University Linz claim they have bested that new record by one step. Further ReadingHow computers got shockingly good at recognizing images
Matrix multiplication, which involves multiplying two rectangular arrays of numbers, is often found at the heart of speech recognition, image recognition, smartphone image processing, compression, and generating computer graphics. Graphics processing units (GPUs) are particularly good at performing matrix multiplication due to their massively parallel nature. They can dice a big matrix math problem into many pieces and attack parts of it simultaneously with a special algorithm.
In 1969, a German mathematician named Volker Strassen discovered the previous-best algorithm for multiplying 44 matrices, which reduces the number of steps necessary to perform a matrix calculation. For example, multiplying two 44 matrices together using a traditional schoolroom method would take 64 multiplications, while Strassen’s algorithm can perform the same feat in 49 multiplications. Enlarge / An example of matrix multiplication from DeepMind, with fancy brackets and colorful number circles.DeepMind
Using a neural network called AlphaTensor, DeepMind discovered a way to reduce that count to 47 multiplications, and its researchers published a paper about the achievement in Nature last week.
Going from 49 steps to 47 doesn’t sound like much, but when you consider how many trillions of matrix calculations take place in a GPU every day, even incremental improvements can translate into large efficiency gains, allowing AI applications to run more quickly on existing hardware.
Advertisement When math is just a game, AI wins EnlargeDeepMind
AlphaTensor is a descendant of AlphaGo (which bested world-champion Go players in 2017) and AlphaZero, which tackled chess and shogi. DeepMind calls AlphaTensor “the “first AI system for discovering novel, efficient and provably correct algorithms for fundamental tasks such as matrix multiplication.”
To discover more efficient matrix math algorithms, DeepMind set up the problem like a single-player game. The company wrote about the process in more detail in a blog post last week:
In this game, the board is a three-dimensional tensor (array of numbers), capturing how far from correct the current algorithm is. Through a set of allowed moves, corresponding to algorithm instructions, the player attempts to modify the tensor and zero out its entries. When the player manages to do so, this results in a provably correct matrix multiplication algorithm for any pair of matrices, and its efficiency is captured by the number of steps taken to zero out the tensor.
DeepMind then trained AlphaTensor using reinforcement learning to play this fictional math gamesimilar to how AlphaGo learned to play Goand it gradually improved over time. Eventually, it rediscovered Strassen’s work and those of other human mathematicians, then it surpassed them, according to DeepMind. Further ReadingNew neural network teaches itself Go, spanks the pros
In a more complicated example, AlphaTensor discovered a new way to perform 55 matrix multiplication in 96 steps (versus 98 for the older method). This week, Manuel Kauers and Jakob Moosbauer of Johannes Kepler University in Linz, Austria, published a paper claiming they have reduced that count by one, down to 95 multiplications. It’s no coincidence that this apparently record-breaking new algorithm came so quickly because it built off of DeepMind’s work. In their paper, Kauers and Moosbauer write, “This solution was obtained from the scheme of [DeepMind’s researchers] by applying a sequence of transformations leading to a scheme from which one multiplication could be eliminated.”
Tech progress builds off itself, and with AI now searching for new algorithms, it’s possible that other longstanding math records could fall soon. Similar to how computer-aided design (CAD) allowed for the development of more complex and faster computers, AI may help human engineers accelerate its own rollout. Promoted Comments Scintillant Seniorius Lurkius et Subscriptor jump to post rbtr4bp wrote:It would be great if you gave some insight in to how you could reduce the numbers of steps for something that is so procedurally defined. Are you saving certain intermediate results? Some other way with determinants? Guessing and being right some of the time while correcting the other times but, on average, faster?
You essentially find specific ways of combining the various matrix values that look weird but save steps. Here’s an example from Deepmind comparing the “textbook” way to multiply 2×2 matrices vs. Strassen’s method. The new algorithms work much the same way, just even more complicated. The algorithms are deterministic, no guessing needed.
Source: Deepmind’s blog post about this research
40 posts | registered 10/17/2013 reader comments 80 with 51 posters participating Share this story Share on Facebook Share on Twitter Share on Reddit Benj Edwards Benj Edwards is an AI and Machine Learning Reporter for Ars Technica. For over 16 years, he has written about technology and tech history for sites such as The Atlantic, Fast Company, PCMag, PCWorld, Macworld, How-To Geek, and Wired. In 2005, he created Vintage Computing and Gaming. He also hosted The Culture of Tech podcast and contributes to Retronauts. Twitter @benjedwards Advertisement
You must login or create an account to comment. Channel Ars Technica ← Previous story Next story → Related Stories Today on Ars