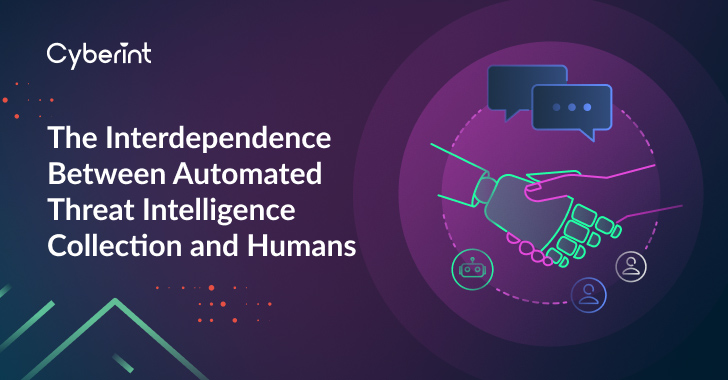
The volume of cybersecurity vulnerabilities is rising, with close to 30% more vulnerabilities found in 2022 vs. 2018. Costs are also rising, with a data breach in 2023 costing $4.45M on average vs. $3.62M in 2017.
In Q2 2023, a total of 1386 victims were claimed by ransomware attacks compared with just 831 in Q1 2023. The MOVEit attack has claimed over 600 victims so far and that number is still rising.
To people working in cybersecurity today, the value of automated threat intelligence is probably pretty obvious. The rising numbers specified above, combined with the lack of cybersecurity professionals available, mean automation is a clear solution. When threat intelligence operations can be automated, threats can be identified and responded to, and with less effort on the part of engineers.
However, a mistake that organizations sometimes make is assuming that once they’ve automated threat intelligence workflows, humans are out of the picture. They conflate automation with completely hands-off, humanless threat intelligence.
In reality, humans have very important roles to play, even (or perhaps especially) in highly automated operations. As Pascal Bornet of Aera Technology puts it, “intelligent automation is all about people,” and automated threat intelligence is no exception.
Automated threat intelligence: A brief history
Threat intelligence wasn’t always automated. It was a reactive process. When an issue arose, the Security Operations Center (SOC) team – or, in certain industries, a fraud team dedicated to collecting intelligence about risks – investigated manually. They searched the dark web for more information about threats, endeavoring to discover which threats were relevant and how threat actors were planning to act.
From there, threat intelligence operations slowly became more proactive. Threat analysts and researchers strove to identify issues before they affected their organizations. This led to predictive threat intelligence, which allowed teams to identify threats before the threat actors were on the fence, trying to get in.
Proactive threat intelligence was not automated threat intelligence, however. The workflows were highly manual. Researchers sought out threat actors by hand, found the forums where they hung out and chatted with them. That approach didn’t scale, because it would require an army of researchers to find and engage every threat actor on the web.
To address that shortcoming, automated threat intelligence emerged. The earliest forms of automation involved crawling the dark web automatically, which made it possible to find issues faster with much less effort from researchers. Then threat intelligence automations went deeper, gaining the ability to crawl closed forums, such as Telegram groups and Discord channels, and other places where threat actors gather, like marketplaces. This meant that automated threat intelligence could pull information from across the open web, the dark web and the deep web (including social channels), making the entire process faster, more scalable and more effective.
Solving the threat intelligence data challenge
Automated threat intelligence helped teams operate more efficiently, but it presented a novel challenge: How to manage and make sense of all the data that automated threat intelligence processes produced.
This is a challenge that arises whenever you collect vast amounts of information. “More data, more problems,” as Wired puts it.
The main issue that teams face when working with troves of threat intelligence data is that not all of it is actually relevant for a given organization. Much of it involves threats that don’t impact a particular business, or simply “noise”– for example, a threat actor discussion about their favorite anime series or what type of music they listen to while writing vulnerability exploits.
The solution to this challenge is to introduce an additional layer of automation by applying machine learning processes to threat intelligence data. In general, machine learning (ML) makes it much easier to analyze large bodies of data and find relevant information. In particular, ML makes it possible to structure and tag threat intel data, then find the information that’s relevant for your business.
For example, one of the techniques that Cyberint uses to process threat intelligence data is correlating a customer’s digital assets (such as domains, IP addresses, brand names, and logos) with our threat intelligence data lake to identify relevant risks. If a malware log contains “examplecustomerdomain.com,” for instance, we’ll flag it and alert the customer. In cases where this domain appears in the username field, it’s likely that an employee’s credentials have been compromised. If the username is a personal email account (e.g., Gmail) but the login page is on the organization’s domain, we can assume that it’s a customer who has had their credentials stolen. The latter case is less of a threat, but Cyberint alerts customers to both risks.
The role of humans in custom threat intelligence
In a world where we’ve fully automated threat intelligence data collection, and on top of that, we’ve automated the analysis of the data, can humans disappear entirely from the threat intelligence process?
The answer is a resounding no. Effective threat intelligence remains highly dependent on humans, for several reasons.
Automation configuration
For starters, humans have to develop the programs that drive automated threat intelligence. They need to configure these tools, improve and optimize their performance, and add new features to overcome new obstacles, such as captchas. Humans must also tell automated collection tools where to look for data, what to collect, where to store it, and so on.
In addition, humans must design and train the algorithms that analyze the data after collection is complete. They must ensure that threat intelligence tools identify all relevant threats, but without searching so broadly that they surface irrelevant information and produce a flood of false positive alerts.
In short, threat intelligence automations don’t build or configure themselves. You need skilled humans to do that work.
Optimizing automations
In many cases, the automations that humans build initially turn out not to be ideal, due to factors that engineers couldn’t predict initially. When that happens, humans need to step in and improve the automations in order to drive actionable threat intelligence.
For example, imagine that your software is generating alerts about credentials from your organization being placed for sale on the dark web. But upon closer investigation, it turns out that they’re fake credentials, not ones that threat actors have actually stolen – so there’s no real risk to your organization. In this case, threat intelligence automation rules would need to be updated to validate the credentials, perhaps by cross-checking the username with an internal IAM system or an employee register, before issuing the alert.
Tracking threat automation developments
Threats are always evolving, and humans need to ensure that strategic threat intelligence tools evolve with them. They must perform the research required to identify the digital locations of new threat actor communities as well as novel attack strategies, then iterate upon intelligence collection tools to keep up with the evolving threat landscape.
For example, when threat actors began using ChatGPT to generate malware, threat intelligence tools needed to adapt to recognize the novel threat. When ExposedForums emerged, human researchers detected the new forum and updated their tools to gather intelligence from this new source. Likewise, the shift to reliance on Telegram by threat actors required threat intelligence tools to be reconfigured to crawl additional channels.
Validating automations
Automations must often be validated to ensure that they’re creating the most relevant information. Large organizations receive tons of alerts, and automated filtering of them only goes so far. Sometimes, a human analyst is needed to go in and evaluate a threat.
For instance, maybe automated threat intelligence tools have identified a potential phishing site that may be impersonating the monitored brand. Perhaps the brand name is in a particular URL, either in a subdomain, the primary domain, or a subdirectory. It might be a phishing site but it could also be a “fan website,” meaning a site created by someone who is paying tribute to the brand (e.g., writing positive reviews, describing favorable experiences with your brand and products, etc.). To tell the difference, an analyst is required to investigate the alert.
Download our guide: The Big Book of the Deep and Dark Web
The benefits and limitations of automated threat intelligence
Automation is a great way to collect threat intelligence data from across the open, deep and dark webs. Automation can be used – in the form of machine learning – to help analyze threat intelligence information efficiently.
But the automation algorithms need to be written, maintained and optimized by humans on an ongoing basis. Humans are also needed to triage alerts, throw out false positives and investigate potential threats. Even with today’s advanced AI solutions, it’s difficult to imagine a world where these tasks can be completely automated in such a way that no human interaction is required. This may be possible in the world of science fiction but it’s certainly not a reality we will see come to fruition in the near future.
Cyberint’s deep and dark web scanning capabilities help to identify relevant risks for organizations, from data leaks and exposed credentials to malware infections and targeted chatter in threat actor forums. Cyberint delivers impactful intelligence alerts, saving teams time by lowering the rate of false positives and accelerating investigation and response processes.
See for yourself by requesting a Cyberint demo.